Predicting the modulus of elasticity of biocompatible titanium alloys using machine learning
Аутори
Marković, Gordana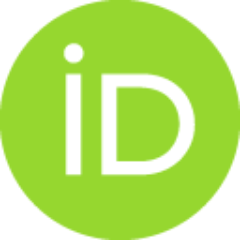
Manojlović, Vaso
Sokić, Miroslav
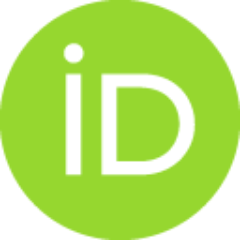
Ruzic, Jovana
Milojkov, Dušan
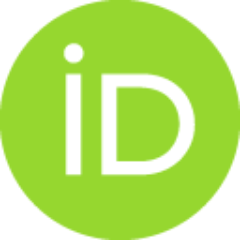
Patarić, Aleksandra
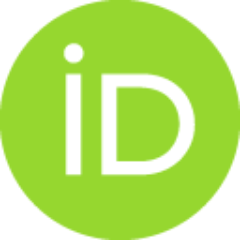
Остала ауторства
Sokić, MiroslavMarković, Branislav
Manojlović, Vaso
Конференцијски прилог (Објављена верзија)
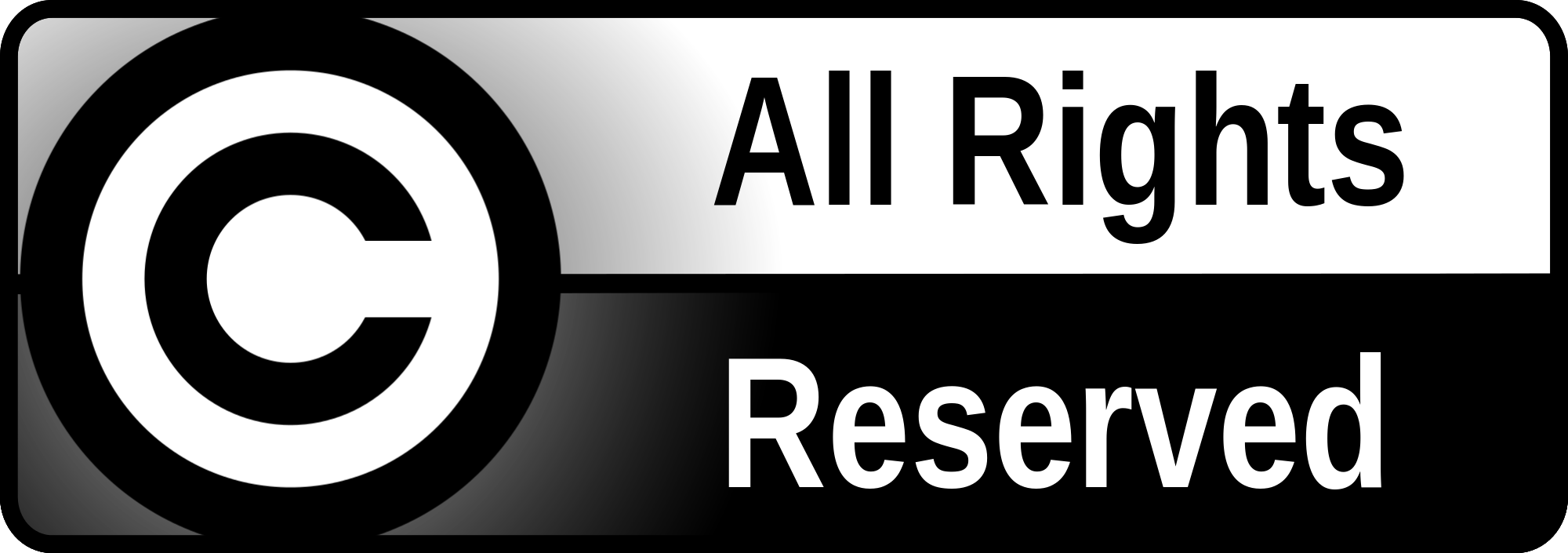
Метаподаци
Приказ свих података о документуАпстракт
Titanium alloys are widely employed in various fields, particularly in biomedical engineering, due to their mechanical and corrosion resistance properties combined with good biocompatibility. The modulus of elasticity is a distinguishing feature of this group of materials compared to others used for similar purposes. A database of approximately 238 titanium alloys free of toxic elements was compiled for this study. The influence of different factors (such as alloy element proportions, density, and specific heat) on the modulus of elasticity was predicted using four methods: support vector machine, XGBoost, Neural Network, and Random Forest. The Random Forest mean absolute error (MAE) of 7.33 GPa, falls within the range of experimentally obtained absolute errors in the literature (up to about 11 GPa). A strong correlation (R2 = 0.72) was observed between experimental and predicted data. Lastly, specific alloying element regions were identified for the modulus of elasticity, which can... be used to design new biocompatible titanium alloys in the future.
Кључне речи:
titanium alloys / modulus of elasticity / biocompatibilityИзвор:
5th Metallurgical & Materials Engineering Congress of South-East Europe, 2023, 154-158Издавач:
- Belgrade : Association of Metallurgical Engineers of Serbia
Финансирање / пројекти:
- Министарство науке, технолошког развоја и иновација Републике Србије, институционално финансирање - 200135 (Универзитет у Београду, Технолошко-металуршки факултет) (RS-MESTD-inst-2020-200135)
- Министарство науке, технолошког развоја и иновација Републике Србије, институционално финансирање - 200023 (Институт за технологију нуклеарних и других минералних сировина - ИТНМС, Београд) (RS-MESTD-inst-2020-200023)
Институција/група
Institut za tehnologiju nuklearnih i drugih mineralnih sirovinaTY - CONF AU - Marković, Gordana AU - Manojlović, Vaso AU - Sokić, Miroslav AU - Ruzic, Jovana AU - Milojkov, Dušan AU - Patarić, Aleksandra PY - 2023 UR - https://ritnms.itnms.ac.rs/handle/123456789/927 AB - Titanium alloys are widely employed in various fields, particularly in biomedical engineering, due to their mechanical and corrosion resistance properties combined with good biocompatibility. The modulus of elasticity is a distinguishing feature of this group of materials compared to others used for similar purposes. A database of approximately 238 titanium alloys free of toxic elements was compiled for this study. The influence of different factors (such as alloy element proportions, density, and specific heat) on the modulus of elasticity was predicted using four methods: support vector machine, XGBoost, Neural Network, and Random Forest. The Random Forest mean absolute error (MAE) of 7.33 GPa, falls within the range of experimentally obtained absolute errors in the literature (up to about 11 GPa). A strong correlation (R2 = 0.72) was observed between experimental and predicted data. Lastly, specific alloying element regions were identified for the modulus of elasticity, which can be used to design new biocompatible titanium alloys in the future. PB - Belgrade : Association of Metallurgical Engineers of Serbia C3 - 5th Metallurgical & Materials Engineering Congress of South-East Europe T1 - Predicting the modulus of elasticity of biocompatible titanium alloys using machine learning EP - 158 SP - 154 ER -
@conference{ author = "Marković, Gordana and Manojlović, Vaso and Sokić, Miroslav and Ruzic, Jovana and Milojkov, Dušan and Patarić, Aleksandra", year = "2023", abstract = "Titanium alloys are widely employed in various fields, particularly in biomedical engineering, due to their mechanical and corrosion resistance properties combined with good biocompatibility. The modulus of elasticity is a distinguishing feature of this group of materials compared to others used for similar purposes. A database of approximately 238 titanium alloys free of toxic elements was compiled for this study. The influence of different factors (such as alloy element proportions, density, and specific heat) on the modulus of elasticity was predicted using four methods: support vector machine, XGBoost, Neural Network, and Random Forest. The Random Forest mean absolute error (MAE) of 7.33 GPa, falls within the range of experimentally obtained absolute errors in the literature (up to about 11 GPa). A strong correlation (R2 = 0.72) was observed between experimental and predicted data. Lastly, specific alloying element regions were identified for the modulus of elasticity, which can be used to design new biocompatible titanium alloys in the future.", publisher = "Belgrade : Association of Metallurgical Engineers of Serbia", journal = "5th Metallurgical & Materials Engineering Congress of South-East Europe", title = "Predicting the modulus of elasticity of biocompatible titanium alloys using machine learning", pages = "158-154" }
Marković, G., Manojlović, V., Sokić, M., Ruzic, J., Milojkov, D.,& Patarić, A.. (2023). Predicting the modulus of elasticity of biocompatible titanium alloys using machine learning. in 5th Metallurgical & Materials Engineering Congress of South-East Europe Belgrade : Association of Metallurgical Engineers of Serbia., 154-158.
Marković G, Manojlović V, Sokić M, Ruzic J, Milojkov D, Patarić A. Predicting the modulus of elasticity of biocompatible titanium alloys using machine learning. in 5th Metallurgical & Materials Engineering Congress of South-East Europe. 2023;:154-158..
Marković, Gordana, Manojlović, Vaso, Sokić, Miroslav, Ruzic, Jovana, Milojkov, Dušan, Patarić, Aleksandra, "Predicting the modulus of elasticity of biocompatible titanium alloys using machine learning" in 5th Metallurgical & Materials Engineering Congress of South-East Europe (2023):154-158.